Quantum-inspired AI
with a shared knowledge base.
eeve.ai is built on an artificial neural network, that is to say, a mathematical model consisting of quantum-inspired artificial ‘neurons’, the qubit, which are able to automatically improve their performances through supervised and unsupervised learning. The shared knowledge base is composed of an extremely innovative data network entailing graphs. Within this structure, the nodes are the concepts/subjects/entities semantically connected with the relationships, which become increasingly more sophisticated during the interactions with the users.
There are three main types of nodes.
Lemma
The lemma, which contains basic pieces of information (e.g., person, cat, vehicle).
Concept
The concept, which includes different lemmas, hence creating a hierarchically higher node (e.g., protected animal).
Inference
An inference includes both lemma and concept nodes allowing for the creation of deductions, which are the result of a deductive process.
Latest Award.
The Global Annual Achievement Awards for Artificial Intelligence Winner of the Best New Topology for AI.
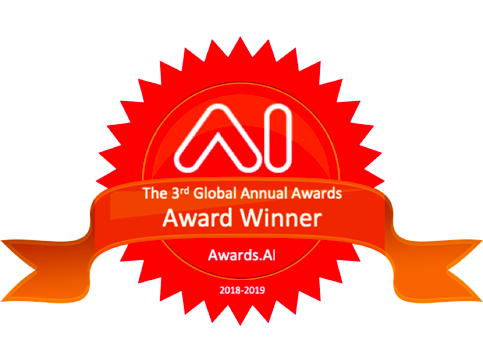
Other Awards.
Eeve.ai is able to combine data-based with algorithm-based structures.
leading to clear advantages when compared to the standard solutions, such as: